George Karniadakis - From PINNs to DeepOnets
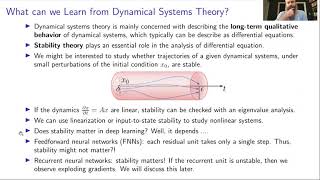
1:09:47
Michael Mahoney - Dynamical systems and machine learning
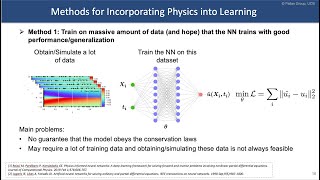
51:22
Rethinking Physics Informed Neural Networks [NeurIPS'21]
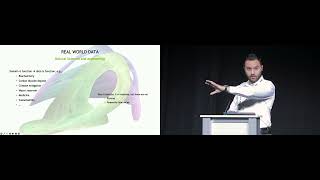
2:06:19
ICML 2024 Tutorial"Machine Learning on Function spaces #NeuralOperators"
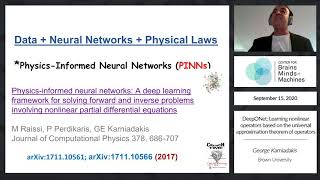
58:12
DeepOnet: Learning nonlinear operators based on the universal approximation theorem of operators.
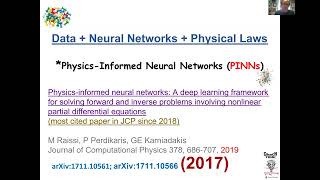
1:03:21
George Karniadakis: Approximating functions, functionals and operators with neural networks
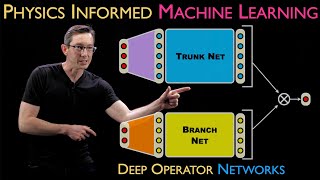
17:17
Deep Operator Networks (DeepONet) [Physics Informed Machine Learning]
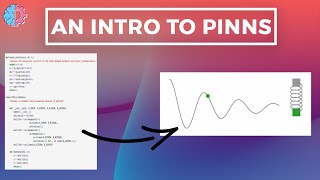
1:10:36
Physics-Informed Neural Networks (PINNs) - An Introduction - Ben Moseley | Jousef Murad
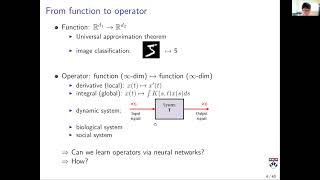
1:11:50